According to Gartner, around 34% of companies use AI in their security tools. This is just the beginning; 56% more organizations are looking forward to getting AI into their security infrastructure. As cyber threats are escalating daily, organizations must have a robust detection system to secure their digital database.
Most CISOs and CIOs are considering integrating AI and ML in threat detection systems, knowing that security crimes have become competent. On the other hand, AI integration involves the use of APIs. These APIs help different software applications to collaborate and exchange data. This enables AI and ML models to integrate into existing security infrastructure, allowing them to analyze vast amounts of security data and identify potential threats in real-time. The process is more complex than it sounds, but if you stay with us, you will quickly grasp it.
Integrating AI and ML In Your Security Infrastructure
Integrating AI and ML with existing security tools and infrastructure involves a strategic and well-thought-out approach. The initial step involves thoroughly assessing the security infrastructure to identify its strengths, weaknesses, and compatibility with AI/ML-based systems.
Once the existing infrastructure has been evaluated, the organization must state what it aims to achieve by incorporating AI and ML-based systems - whether enhancing threat detection, improving incident response times, or automating routine security tasks. This clarity in objectives serves as a guiding principle throughout the integration journey.
The subsequent step is selecting AI/ML solutions that align with integration goals and are compatible with the existing infrastructure. Next, you must use Application Programming Interfaces (APIs) because they bridge the gap, facilitating easy communication between diverse security tools and AI/ML systems. Making sure you have well-documented APIs that support necessary data exchange is paramount.
Now, you have to normalize data formats across security tools to ensure uniformity, allowing the AI/ML system to analyze and interpret the data it receives effectively. Then, rigorous integration testing follows to identify and address potential issues before full deployment, testing for interoperability, data accuracy, and system performance.
Role Of APIs As Facilitators
APIs serve as the linchpin, facilitating communication and interaction between disparate systems, ultimately enabling a harmonized cybersecurity ecosystem. Regarding AI and ML solution integration, API acts as a bridge to connect the newly introduced systems with the existing security infrastructure. These interfaces standardize communication protocols, allowing data to flow between security architecture components. Well-designed APIs streamline the integration process, ensuring that AI/ML systems can effectively utilize data generated by security tools for analysis and decision-making for threat detection.
APIs are amazingly interoperable; they break down silos within the security infrastructure. This interoperability is fundamental for achieving a cohesive and efficient cybersecurity framework where AI and ML collaborate with established security tools. Moreover, APIs facilitate flexibility in the integration process. They allow organizations to adopt new AI/ML solutions without overhauling their security architecture.
Impact Of Integration on Network Performance
This integration can discernably impact network performance, necessitating careful consideration of the trade-offs involved. This integration causes an additional computational load imposed by AI and ML algorithms. These algorithms require substantial processing power to analyze large volumes of data in real time, potentially leading to latency or slower response times. That's why we suggest you assess the capacity of the existing network to handle the augmented processing requirements without compromising performance.
Another aspect influencing network performance is the data transfer between security tools and the AI/ML systems. The integration necessitates a seamless flow of data for analysis, and this increased data exchange can result in higher network traffic. Organizations must evaluate their network bandwidth to ensure it can accommodate the augmented data transfer without causing bottlenecks or degrading the overall network performance.
Are AI and ML Solutions Scalable?
Integrating AI and ML with security tools and infrastructure can have scalability concerns. Organizations are using these advanced technologies to strengthen their cybersecurity, while the ability to adapt and grow in response to evolving demands becomes a critical factor for long-term success.
Scalability refers to the system's capability to handle increasing volumes of data and computational complexity without sacrificing performance. As cyber threats evolve, the integrated solution must be able to scale to accommodate larger datasets, heightened analysis requirements, and the expanding scope of security responsibilities.
An essential consideration is the scalability of AI and ML algorithms themselves. The chosen algorithms should be effective in current scenarios and capable of scaling to address future, more complex threats. This requires careful selection and continuous refinement of algorithms to ensure they remain relevant and effective as the organization's cybersecurity needs evolve.
Training And Educating Employees
These advanced technologies generate complex insights, and using the security team to understand and use the data is essential for optimizing the capabilities of the integrated system.
Companies must consider conducting a training program with a comprehensive introduction to the AI and ML models integrated into the cybersecurity framework. This foundational knowledge provides employees with a clear understanding of how these technologies operate, the types of data they analyze, and the insights they generate. This understanding forms the basis for effective data interpretation.
Subsequently, training should focus on developing the analytical skills required to interpret the output of AI and ML algorithms. This includes identifying patterns, anomalies, and potential threats within the data. Practical exercises, case studies, and simulated scenarios can enhance these analytical skills, allowing employees to apply theoretical knowledge to real-world situations.
Choose Solutions That Provide Vendor Support
The collaboration between organizations and solution providers helps navigate the complexities of integration and ensures an effective cybersecurity ecosystem.
Effective communication channels with vendors throughout the integration help in open dialogue, allowing organizations to seek clarification, address concerns, and obtain timely assistance as needed. Access to responsive support teams ensures that any unexpected issues can be promptly resolved, preventing disruptions to the cybersecurity infrastructure.
Vendor support extends beyond the integration phase to encompass ongoing maintenance and updates. Regular software updates, patches, and enhancements are critical to keeping the integrated system resilient against evolving threats. Vendors' commitment to continuous support demonstrates their dedication to the long-term success of their solutions within the organization.
We at PRODAFT are fully aware of these commitments and a supportive environment. Our clients have the 24/7 support of our threat intelligence analysts, making it easy to address any additional queries or concerns. Our goal is to ensure better efficiency with less workload, making the U.S.T.A. platform the perfect solution for cybersecurity teams across all verticals.
Final Thoughts
Organizations should invest in technologies that facilitate rapid response and containment. Automated response mechanisms, coupled with AI-driven analytics, enable organizations to detect and mitigate threats in near real-time, reducing the potential impact of emerging cybersecurity threats.
Adapting to emerging threats requires a multifaceted approach that includes agile AI/ML models, continuous threat intelligence monitoring, employee training, collaborative efforts, robust testing, and a well-defined incident response plan. By embracing a proactive and comprehensive strategy, organizations can effectively navigate the ever-changing cybersecurity landscape and maintain a resilient defense against emerging threats.
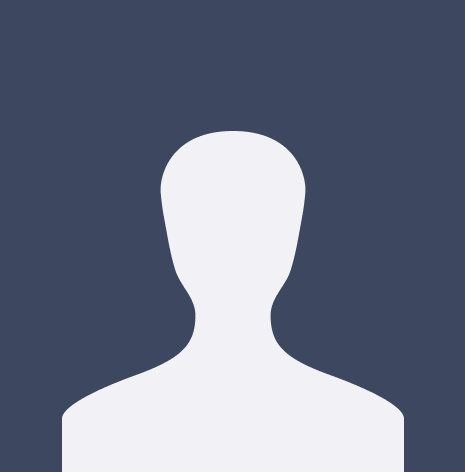
PRODAFT Team
Stay up to date
Browse Posts
Browse by topics
- Cybercrime awareness (15)
- Cybersecurity for Businesses (10)
- Risk management (7)
- Cybersecurity Tips (6)
- Digital safety (6)
- Proactive Threat Intelligence (6)
- Cyber threat protection (5)
- Cyberattacks (5)
- Risk intelligence (5)
- Threat Prevention (5)
- Data Protection (4)
- Network Security (4)
- Phishing (4)
- Supply chain risks (4)
- TTPs (4)
- Artificial intelligence (3)
- Critical network infrastructures (3)
- Malicious websites (3)
- Malware (3)
- Social engineering (3)
- Data breaches (2)
- Insider Threats (2)
- NIS2 Directive (2)
- Ransomware (2)
- CISOs (1)
- Cryptocurrencies (1)
- Cyber espionage (1)
- Cyber extortion (1)
- Cyber fraud (1)
- Cybersecurity Collaboration (1)
- DDoS Attacks (1)
- Deepfakes (1)
- DoS Attacks (1)
- Fake social media (1)
- Incident Response (1)
- Internet of things (1)
- Money Laundering (1)
- Multi-factor authentication (1)
- One-day vulnerability (1)
- Remote Work (1)
- Stealers (1)
- SystemBC (1)
- The Cyber kill chain (1)
- Threat Intelligence solutions (1)
- Tor browser (1)
- Traffic Distribution System (1)
- Zero-day vulnerability (1)
- dark web (1)
- deep web (1)
- keyloggers (1)
- social media (1)
- spoofing (1)
- threat detection (1)